Review Article
Connected Health Apps and Devices: Implications for Healthcare Delivery
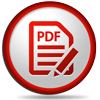
Helen Figge1* and Joseph C Kvedar2
1Adjunct Professor, Global Healthcare Management, MCPHS University, USA
2Professor of Dermatology, Harvard Medical School, USA
*Address for Correspondence: Helen Figge, BS, PharmD., MBA, CPHIMS, FHIMSS, Adjunct Professor, Global Healthcare Management, MCPHS University, Chief Strategy Officer, MedicaSoft, Senior Advisor, National Health IT (NHIT) Collaborative for Underserved, New York HIMSS, National Liaison, Health 2.0 Boston, Past Chair, Chair Innovation, USA, Tel: 518-727-6234; Email: helenlf01@gmail.com
Dates: Submitted: 15 June 2019; Approved: 24 June 2019; Published: 25 June 2019
How to cite this article: Figge H, Kvedar JC. Connected Health Apps and Devices: Implications for Healthcare Delivery. Clin J Nurs Care Pract. 2019; 3: 035-039. DOI: 10.29328/journal.cjncp.1001014
Copyright License: © 2019 Figge H, et al. This is an open access article distributed under the Creative Commons Attribution License, which permits unrestricted use, distribution, and reproduction in any medium, provided the original work is properly cited.
Keywords: Digital health; Wearable computing; Telehealth; Remote patient monitoring
Abstract
Healthcare is realizing the importance of health information technology - its applications and devices in play today. Advancing healthcare best practices will rely on up to date data and analysis to provide the most effective forms of therapy. Healthcare is becoming more reliant on patients who are engaging in their own healthcare. With this we are seeing an increase in available health related apps for these devices. This summary reviews various connected health strategies using proven apps and devices to improving the quality of care, promoting patient engagement, and improving outcomes. Here we discuss several trends and the healthcare delivery implications.
Review
The widespread adoption of connected and digital health technologies and health-related apps are empowering patients to monitor their health [1,2]. Some view these technologies as a key approach to improving quality of care, patient engagement, and outcomes [3]. The addition of genetic information to the mix promises to enable more personalized options for the diagnosis and management of chronic disease. Hence, the locus of care will potentially move from the doctor’s office and hospital to where the patient is, creating a number of important changes to care delivery. Here we discuss several trends and the healthcare delivery implications.
Weight and cardiovascular risk factor management
Controlling obesity -- a risk factor for chronic illnesses, including type II diabetes and cardiovascular disease, conditions known to be responsive to increased physical activity -- is a high-priority public health goal [4]. Between 2011 to 2017, there were an estimated 423 unique wearable devices on the market for weight management, including fitness trackers and smart watches [5]. Websites and smartphone apps can also help evaluate caloric intake, and “smart scales” can synch with software applications to provide consumers continuous feedback. Evidence demonstrating improved health outcomes is accumulating, especially when these devices are combined with behavioral interventions [6-10]. However, in less motivated populations with pre-diabetes or diabetes, these strategies might not be effective, and more innovative approaches, and additional research, is still needed [11]. Mobile health (mHealth) technologies can give primary care practices and patient-centered medical homes important opportunities to partner with patients. For example, individuals not making acceptable progress with weight reduction can be quickly identified and a case manager or clinician alerted to trigger a coaching call. Patient-generated data can be incorporated into the patient’s chart for periodic review along with other clinical data, such as lab results. By capturing data from consumer wearables and displaying these data in the context of the electronic record, we can optimize clinician and patient engagement.
The Centers for Medicare and Medicaid Services (CMS) recently approved new reimbursement codes for remote monitoring of chronic illness. Providers must integrate these codes into revenue cycle operations. Training clinical staff (nurses and mid-level providers) to check incoming data from patients and escalate decision making to a physician, as needed, is another important step.
Diabetes management
Wearable continuous glucose monitors (CGMs) and flash glucose monitoring devices (a wearable sensor and smartphone/reader to scan the sensor), including the DEXCOM G5/G6 and the Freestyle Libre Sensor, promise to revolutionize diabetes management. These devices enable tight glycemic control while minimizing hyper- and hypoglycemic episodes, which can result in improved HbA1c and “time in-control” for patients with both type I and type II diabetes [12]. Patients using approved CGMs can self-adjust insulin doses without confirming results with capillary glucose (fingerstick) readings [13]. The FDA evaluated data from 2 clinical studies including 324 adults and children in which DEXCOM G6 readings were compared against laboratory measurements of blood glucose, and found no serious adverse events [14]. Several landmark trials have demonstrated the efficacy of CGM technology in patients requiring multiple daily insulin injections (MDI) [15], continuous subcutaneous insulin infusion (CSII) [16], and in patients with hypoglycemic unawareness [17]. In the future, we expect machine learning and robust artificial intelligence (AI) will assist with this type of analysis. One emerging example is the “applied health signals engine” from Livongo [18]. CGM systems are now being deployed in closed-loop configurations with CSII; the Medtronic Minimed 670G system approved by the U.S. Food and Drug Administration (FDA) is on the market [19]. Others are likely to soon follow.
mHealth technologies will enable providers to partner with patients with diabetes, such as adjusting treatment regimens via the patient’s mobile device. AI software can efficiently evaluate patient-generated health data from mHealth tools, along with lab results (e.g., glucose levels, renal function, HbA1c, and lipid profiles) and eye exam reports, to continuously monitor patients’ progress. Patients needing more intensive intervention can meet with a certified diabetes educator or have a scheduled clinic visit. These technologies allow for implementation of population health goals, and makes patient management truly time- and place-independent.
Recommendations for practice readiness, similar to those for weight management, include additional emphasis on integrating home glucose monitoring data into sections of the electronic record where clinicians can easily view patients’ relevant lab data.
Congestive Heart Failure (CHF) management
This chronic condition consumes significant healthcare resources and is associated with repeated ER visits and hospitalizations, and high rates of readmission. In the United States, CHF is one of the Medicare readmission penalty diseases; the average cost of a single hospitalization for a Medicare recipient is $14,631, with a median length-of-stay of five days [20]. Data demonstrate that 40.2% of Medicare patients with CHF are readmitted within 90 days of a prior hospital discharge [20].
Several studies have shown that home telemonitoring, including digital scales, blood pressure monitoring via smartphones, and pulse oximeters can reduce both hospital admissions and mortality [21-25]. One meta-analysis of home telemonitoring [21], documented a 40%-46% reduction (not statistically significant) in CHF-related admissions, a significant reduction in mortality (30%-67%), and a 53%-62% reduction in bed days. A study from Italy, [22] comparing home telemonitoring with usual care, demonstrated a highly significant reduction in the composite endpoint of mortality and rate of hospitalization. Another meta-analysis [23] including 21 studies (3082 patients), using a random-effects model, demonstrated that home telemonitoring reduced mortality (risk ratio = 0.64; 95% CI: 0.48-0.85) compared with usual care. Several of the studies analyzed in the meta-analysis suggested reduction in hospitalizations. A 20% reduction in all-cause mortality and 21% reduction in rate of hospitalization were documented in a third meta-analysis of 4264 patients [24]. However, another recent meta-analysis suggested that a decline mortality is short-lived; home telemonitoring reduced all-cause and heart failure-related mortality at 180 days, but not at 365 days [25].
In the future, deep machine learning and AI can assess a broader array of clinical and patient-generated data to identify patients requiring more intensive intervention from a nurse or other clinician, improve outcomes and further reduce the cost of care. Furthermore, AI applications can analyze data in an EMR (“EMR crawlers”) and suggest treatment optimizations.
For CHF, practice readiness is a more high-touch intervention than those discussed earlier, and requires coordination between primary care, cardiology and, often, home care. Telemonitoring staff, often nurses in a call center environment, must have shared protocols with contingency orders, as well as clear and open communication with providers managing the patient. When clinically meaningful changes are detected, the care team must act quickly to avoid unnecessary emergency room visits and hospitalizations.
Cardiac Arrhythmia (Atrial Fibrillation) detection and management
Timely detection of atrial fibrillation (A Fib), a known risk factor for stroke, can be facilitated by FDA-approved apps on mobile devices, and is crucial to prevent strokes.
In the REHEARSE-AF study [26], A Fib was more likely to be diagnosed in patients age 65 and older using twice-a-week screening with an AliveCor Kardia monitor attached to a WiFi-enabled iPod than in the usual care group (hazard ratio, 3.9; 95% confidence interval=1.4-10.4; P=0.007). Another recent study demonstrated that the wearable ECG monitoring patch, ZIO XT Patch from iRhythm Technologies, resulted in significantly higher A Fib detection rates, higher rates of anticoagulation, and a greater number of cardiology visits in actively monitored patients, compared to controls where monitoring was delayed [27]. This patch can be mailed to the patient, and self-applied; after monitoring is complete, the patch is mailed to a facility that analyzes the recorded data.
Janssen Pharmaceuticals and Apple recently announced they are collaborating to test an Apple Watch app to detect A Fib [28]. Apple recently released its own app, but had problems with false positive results, compared to a wearable patch [29].
The biggest challenge is integrating technologies offering continuous feedback into a clinical practice model based on episodic care delivery. Who is responsible to identify a positive signal and take appropriate action? Similar to the chronic care management approach above, A Fib often demands more acute management than hypertension, obesity and the like.
Incorporating personal genetic information
Overlaying personal genetic information with mHealth data has the potential to revolutionize chronic disease diagnosis and management. Leveraging AI solutions to analyze a wide array of genetic information, together with other clinical data, mHealth can present the patient and care team with the most appropriate -- and personalized -- treatment options. The combination of genetic data with personalized phenotypic data (from apps and wearables) will enable truly individualized treatment regimens.
Personalized medicine can be enhanced with specific knowledge of disease-causing genetic variants, and greater understanding of how the genomic environment influences individual responses to therapeutic agents [30]. Several marketplace solutions currently offer direct-to-consumer DNA sequencing of the 20,000+/- protein-coding human genes, for under $100 USD [31]. Challenges abound, from how to deal with the vast data stores created, to setting pricing for “N of one” treatments.
Conclusion and Future Vision
mHealth devices and connected health strategies offer a powerful approach to implementing personalized health strategies, creating unprecedented opportunities to improve the diagnosis and management of chronic conditions, and empowering patients to engage in their health and wellness. We believe that the overlay of genetic data with mHealth, deep machine learning, and AI could lead to unparalleled advances in more personalized management of chronic diseases. There will undoubtedly be significant upfront costs and implementation challenges to embed advanced technologies such as AI into the current infrastructure, but the potential for improved quality of care at lower cost will likely ultimately offset the initial costs. Emerging technologies and innovations will need to be carefully vetted with robust, high-quality clinical validation studies to ensure they improve outcomes.
References
- GO-Gulf.com. Smartphone users around the world – statistics and facts [infographic]. 2018; Ref.: http://bit.ly/2LbfW1L
- International Telecommunications Union. The world in 2011: ICT facts and figures. 2011. Retrieved on 12/14/2018; Ref.: http://bit.ly/2ZQy1Gt
- Kvedar J, Coye MJ, Everett W. Connected health: a review of technologies and strategies to improve patient care with telemedicine and telehealth. Health Aff (Millwood). 2014; 33: 194-199. Ref.: http://bit.ly/2Xw7mAZ
- National Institute of Diabetes, and Digestive and Kidney diseases. Health Risks of Being Overweight. Retrieved on 12/14/2018; Ref.: http://bit.ly/2x9wS0g
- Henriksen A, Haugen Mikalsen M, Woldaregay AZ, Muzny M, Hartvigsen G, et al. Using fitness trackers and smartwatches to measure physical activity in research: analysis of consumer wrist-worn wearables. J Med Internet Res. 2018; 20: e110. Ref.: http://bit.ly/2FsvslV
- Shuger SL, Barry VW, Sui X, McClain A, Hand GA, et al. Electronic feedback in a diet- and physical activity-based lifestyle intervention for weight loss: a randomized controlled trial. Int J Behav Nutr Phys Act. 2011; 8: 41. Ref.: http://bit.ly/31Lddlk
- Pellegrini CA, Verba SD, Otto AD, Helsel DL, Davis KK, et al. The comparison of a technology-based system and an in-person behavioral weight loss intervention. Obesity. 2012; 20: 356-363. Ref.: http://bit.ly/2IHZp3D
- Steinberg DM, Tate DF, Bennett GG, Ennett S, Samuel-Hodge C, et al. The efficacy of a daily self-weighing weight loss intervention using smart scales and email. Obesity. 2013; 21: 1789-1797. Ref.: http://bit.ly/2ZFacRI
- Spring B, Duncan JM, Janke E, Kozak AT, Gene McFadden H, et al. Integrating technology into standard weight loss treatment: A randomized controlled trial. JAMA Intern Med. 2013; 173: 105-111. Ref.: http://bit.ly/2xbvVob
- Ross KM, Wing RR. Impact of Newer Self-Monitoring Technology and Brief Phone-Based Intervention on Weight Loss: A Randomized Pilot Study. Obesity. 2016; 24: 1653-1659 Ref.: http://bit.ly/2RBM0gf
- Polgreen LA, Anthony C, Carr L, Simmering JE, Evans NJ, et al. The effect of automated text messaging and goal setting on pedometer adherence and physical activity in patients with diabetes: A randomized controlled trial. PLoS ONE. 13: e0195797. Ref.: http://bit.ly/2xdROTM
- Rodbard D. Continuous Glucose Monitoring: A Review of Recent Studies Demonstrating Improved Glycemic Outcomes. Diabetes Technol Ther. 2017; 19(Suppl 3): S-25–S-37. Ref.: http://bit.ly/2X5ig1m
- US Food and Drug Administration. Press announcement. FDA expands indication for continuous glucose monitoring system, first to replace fingerstick testing for diabetes treatment decisions. 2016; Ref.: http://bit.ly/2FySRm2
- US Food and Drug Administration. Press announcement. FDA authorizes first fully interoperable continuous glucose monitoring system, streamlines review pathway for similar devices. 2018; Ref.: http://bit.ly/2J5vz8g
- Beck RW, Riddlesworth T, Ruedy K, Ahmann A, Bergenstal R, et al. Effect of Continuous Glucose Monitoring on Glycemic Control in Adults with Type 1 Diabetes Using Insulin Injections: The DIAMOND Randomized Clinical Trial. JAMA. 2017; 317: 371–378. Ref.: http://bit.ly/2IHnt6Q
- El-Laboudi AH, Godsland IF, Johnston DG, Oliver NS. Measures of glycemic variability in type 1 diabetes and the effect of real-time continuous glucose monitoring. Diabetes Technol Ther. 2016; 18: 806–812. Ref.: http://bit.ly/31Lebhs
- van Beers CAJ, de Wit M, Kleijer SJ, Geelhoed-Duijvestijn PH, DeVries JH, et al.: Continuous glucose monitoring for patients with type 1 diabetes and impaired awareness of hypoglycaemia (IN CONTROL): a randomized, open-label, crossover trial. Lancet Diabetes Endocrinol. 2016; 4: 893–902. Ref.: http://bit.ly/2X3jHbC
- Accessed on 1/26/2019. Ref.: http://bit.ly/2Y7CfZI
- Bergenstal RM, Garg S, Weinzimer SA, Buckingham BA, Bode BW, et al. Safety of a hybrid closed-loop insulin delivery system in patients with type 1 diabetes. JAMA. 2016; 316: 1407–1408. Ref.: http://bit.ly/2xfdfDW
- Kilgore M, Patel HK, Kielhorn A, Maya JF, Sharma P. Economic burden of hospitalizations of Medicare beneficiaries with heart failure. Risk Manag Health Policy. 2017; 10: 63–70. Ref.: http://bit.ly/2Xw8bK5
- Dang S, Dimmick S, Kelkar G. Evaluating the evidence base for the use of home telehealth remote monitoring in elderly with heart failure. Telemed J E Health. 2009; 15: 783–796. Ref.: http://bit.ly/2RxzvT2
- Antonicelli R, Testarmata P, Spazzafumo L, Gagliardi C, Bilo G, et al. Impact of telemonitoring at home on the management of elderly patients with congestive heart failure. J Telemed Telecare. 2008; 14: 300–305. Ref.: http://bit.ly/2KINzsr
- Polisena J, Tran K, Cimon K, Hutton B, McGill S, Palmer K, et al. Home telemonitoring for congestive heart failure: a systematic review and meta-analysis. J Telemed Telecare. 2010; 16: 68–76. Ref.: http://bit.ly/2J5CnTc
- Clark RA, Inglis SC, McAlister FA, Cleland JG, Stewart S. Telemonitoring or structured telephone support programs for patients with chronic heart failure: systematic review and meta-analysis. BMJ. 2007; 334: 942. Ref.: http://bit.ly/31VAi4K
- Pekmezaris R, Torez L, Willimas M et al. Home telemonitoring in heart failure: a systematic review and meta-analysis. Health Affairs. 2018; 37: 1983-1989. Ref.: http://bit.ly/31USPOU
- Halcox JPJ, Wareham K, Cardew A, Gilmore M, Barry JP, et al. Assessment of Remote Heart Rhythm Sampling Using the AliveCor Heart Monitor to Screen for Atrial Fibrillation: The REHEARSE-AF Study. Circulation. 2017; 136: 1784-1794 Ref.: http://bit.ly/2KIOYzd
- Steinhubl SR, Waalen J, Edwards AM, Ariniello LM, Mehta RR, et al. Effect of a home-based wearable continuous ecg monitoring patch on detection of undiagnosed atrial fibrillation. The mSToPS randomized clinical trial. JAMA. 2018; 320: 146-155. Ref.: http://bit.ly/2ZNUP9U
- Chin K. Johnson & Johnson to use Apple Watch app for heart-health study. The Wall Street Journal. 2019. Ref.: https://on.wsj.com/2LcAh6N
- Rowland C. Apple now says its smartwatch tech to detect atrial fibrillation is not for those with atrial fibrillation. The Washington Post. 2018; Ref.: https://wapo.st/2KDVNSv
- Grishin D, Obbad K, Estep P, Quinn K, Zaranek SW, et al. Accelerating genomic data generation and facilitating genomic data access using decentralization, privacy-preserving technologies and equitable compensation. Blockchain in Healthcare Today. 2019; Ref.: http://bit.ly/2FwuWn9
- Mullin E. A DNA app store is here, but proceed with caution. MIT Technology Review. 2017; Ref.: http://bit.ly/2LmWrn5